AI Offers Hope for Accurate Gallbladder Cancer Detection
Written by Jaskiran Walia, Arushi Sharma
Artificial intelligence (AI) offers hope for accurate gallbladder cancer detection, as an AI model (GBC-net) has been proposed to differentiate benign from malignant gallbladder diseases.
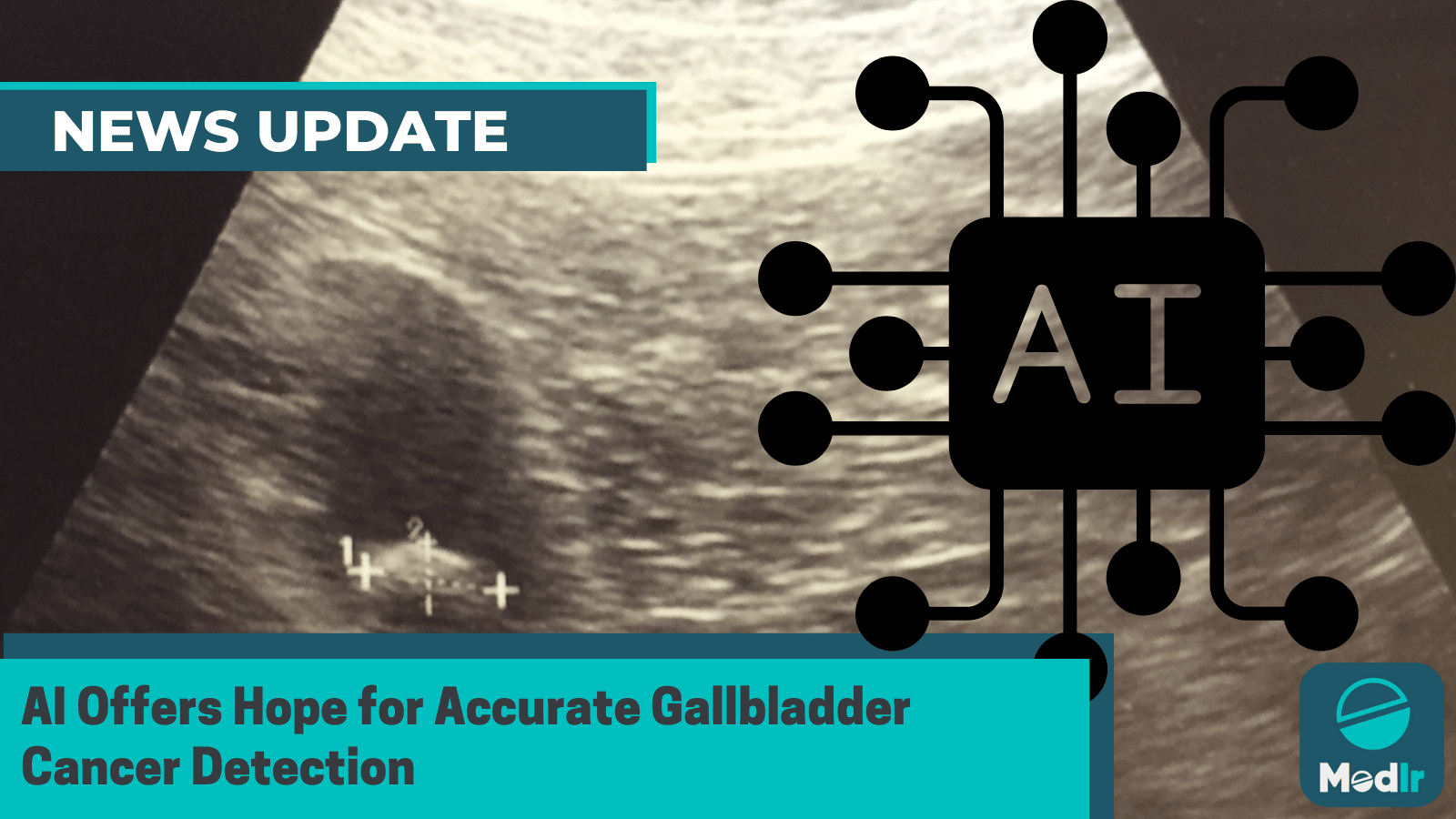
Gallbladder cancer (GBC) is a deadly cancer with a low early detection rate and a high mortality rate.
According to a study published in the journal The Lancet Regional Health - Southeast Asia, an AI system developed by researchers from the Postgraduate Institute of Medical Education and Research (PGIMER) in Chandigarh and the Indian Institute of Technology (IIT) in New Delhi demonstrated diagnostic performance comparable to experienced radiologists in GBC detection.
This AI model, which was trained on 233 patients' abdominal ultrasound data, validated on 59 patients, and tested on 273 patients, shows promise for more accurate early diagnosis of GBC, addressing a critical healthcare challenge.
During the testing phase, two independent radiologists evaluated ultrasound images and compared their diagnostic performance to the DL model. The DL model had a sensitivity of 92.3%, a specificity of 74.4%, and an AUC of 0.887 for GBC detection, which was very close to the performance of the radiologists.
The DL approach had high sensitivity and AUC in detecting GBC, even in cases with stones, contracted gallbladders, small lesions (less than 10 mm), and neck lesions, which was consistent with radiologists' performance.
Notably, the DL model outperformed one radiologist in identifying the mural thickening type of GBC, albeit with slightly lower specificity, according to the study.
“The DL-based approach demonstrated diagnostic performance comparable to experienced radiologists in detecting GBC using ultrasound,” the authors of the study noted.
“Further multicentre studies are recommended to fully explore the potential of DL-based GBC diagnosis,” they added.
The study has acknowledged certain limitations. It relies on a dataset from a single center, and broader validation through multicenter studies is required.
Additionally, the study’s knowledge is limited to data up to 2021, and any advancements in DL and GBC diagnosis beyond that point are not considered.